Unlocking the Power of Image Annotation for Machine Learning: The Fundamental Step in Advanced AI Development
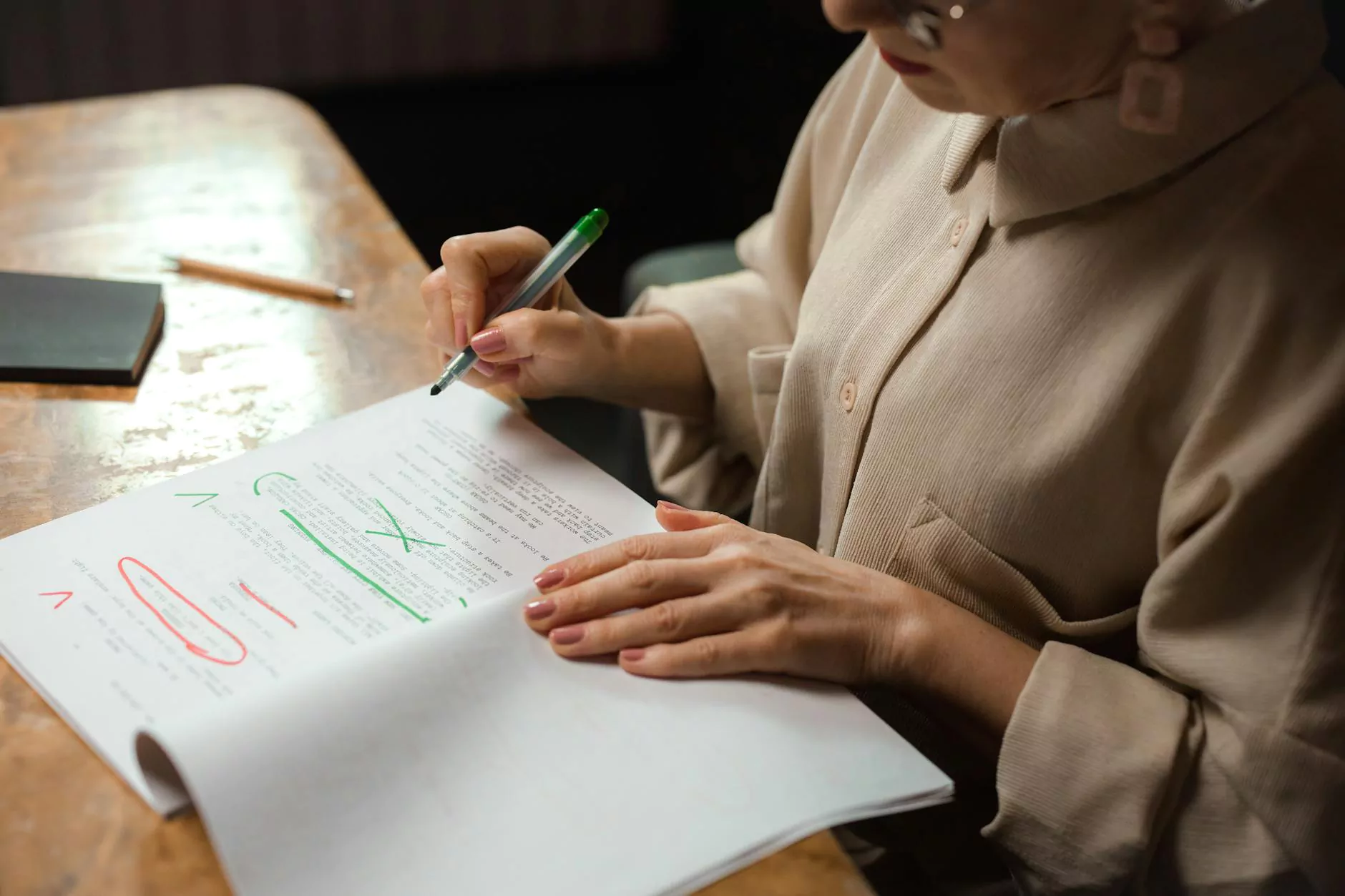
In the rapidly evolving landscape of artificial intelligence and machine learning, the importance of precise and reliable data cannot be overstated. Image annotation for machine learning plays a pivotal role in training models that are not only accurate but also capable of generalizing across varied real-world scenarios. As businesses increasingly seek competitive advantages through AI-driven insights, understanding the nuances of image annotation and its integration into software development becomes essential for success.
Understanding the Role of Image Annotation in Machine Learning
To fully grasp the significance of image annotation for machine learning, it is vital to understand what image annotation entails. It involves the process of labeling images with relevant information, such as bounding boxes, polygons, semantic segmentation, keypoints, and more. This annotated data serves as the foundational training set for machine learning algorithms, enabling them to identify and interpret visual patterns accurately.
Why Is Image Annotation Critical for AI and Machine Learning?
- Enhances Model Accuracy: High-quality annotations provide the precise labels needed for supervised learning models to distinguish between different objects and scenarios effectively.
- Enables Complex Visual Recognition Tasks: Tasks such as autonomous driving, medical imaging diagnosis, retail analytics, and surveillance require detailed annotations that capture nuanced visual details.
- Reduces Error Margins: Well-annotated datasets minimize the risk of misclassification, ensuring that AI outputs are reliable and trustworthy.
- Speeds Up Model Development: Efficient annotation processes accelerate the training cycle, allowing for faster deployment of AI solutions.
The Evolution of Image Annotation Technologies and Techniques
As the demand for sophisticated AI models grows, so does the complexity and variety of image annotation techniques. Traditional manual annotation, while precise, is labor-intensive and not scalable for large datasets. Today, innovative software development in this domain involves semi-automated annotation tools, AI-assisted labeling, and cloud-based annotation platforms that facilitate collaboration and enhance productivity.
Traditional Manual Annotation
This method involves human annotators meticulously labeling each image. While it guarantees accuracy, it is often costly and time-consuming, especially for datasets containing millions of images.
AI-Assisted Annotation
Modern solutions leverage pre-trained models to generate initial annotations, which humans then review and correct. This hybrid approach significantly reduces labeling time while maintaining high accuracy.
Automated and Semi-Automated Tools
Advanced software platforms integrate machine learning algorithms capable of automatic annotations, allowing for scalable and efficient data labeling. These tools include features like smart suggestion, quality checks, and easy correction mechanisms, crucial for large-scale deployments.
The Business Impact of High-Quality Image Annotation in Software Development
Integrating top-tier image annotation for machine learning into software development strategies yields numerous competitive advantages:
- Improved AI Model Performance: Well-annotated data directly translates into more accurate predictive models, which are valuable for predictive analytics, automation, and decision-making.
- Cost Effectiveness: Streamlined annotation processes reduce development costs, optimize resource allocation, and minimize rework due to erroneous labels.
- Faster Time to Market: Faster data preparation accelerates development cycles, allowing businesses to deploy AI-powered products more quickly.
- Enhanced Customer Satisfaction: Accurate models lead to better user experiences, higher engagement, and increased trust in AI products.
Traits of Leading Software Solutions for Image Annotation
In the realm of software development, key features distinguish effective image annotation platforms, including:
- Intuitive User Interface: Simplifies complex labeling tasks and reduces training time for annotators.
- Annotation Flexibility: Supports various annotation types such as bounding boxes, polygons, line annotations, and pixel-level segmentation.
- Quality Control Mechanisms: Integrates review workflows, consensus tools, and validation features to ensure labeling accuracy.
- Integration Capabilities: Easily connects with machine learning pipelines, cloud services, and data storage systems.
- Automation and AI Assistance: Incorporates semi-automated labeling tools powered by AI, boosting efficiency and scale.
Best Practices for Effective Image Annotation in AI Projects
Achieving high-quality annotations requires adherence to best practices, including:
- Clear Annotation Guidelines: Establish explicit instructions to ensure consistency across annotators and datasets.
- Regular Training and Feedback: Continuously improve annotator skills through feedback and training sessions.
- Utilize Advanced Annotation Tools: Leverage software solutions that facilitate efficiency and accuracy.
- Implement Quality Assurance Measures: Conduct periodic reviews and validation checks to maintain labeling standards.
- Scalable Data Management: Use cloud-based platforms that support collaboration, version control, and large data storage.
The Future of Image Annotation in Machine Learning and Software Development
The landscape of image annotation for machine learning is poised for transformative growth. Emerging trends include:
- Automated Annotation Technologies: Continued development of AI-powered tools that minimize human intervention.
- Domain-Specific Labeling Solutions: Customized annotation solutions designed for specific industries like healthcare, automotive, and retail.
- Integrated Data Labeling and Model Training Platforms: Unified environments that streamline data annotation, model development, and deployment.
- Enhanced Collaboration and Crowd-sourcing: Leveraging distributed teams and crowd-sourcing platforms for scalable labeling efforts.
- Semantic and Context-Aware Annotation: Incorporating context and semantic understanding to improve label quality and AI comprehension.
Choosing the Right Partner for Software Development in Image Annotation
To fully harness the benefits of image annotation for machine learning, partnering with experienced and innovative software development providers is crucial. Consider factors such as:
- Expertise in Data Labeling Platforms: Proven track record in building and customizing annotation tools tailored to your needs.
- Integration with AI and ML Pipelines: Ability to seamlessly connect annotation tools with your existing infrastructure.
- Scalability and Performance: Support for large datasets without compromising speed or quality.
- Customer Support and Training: Ongoing assistance to optimize your annotation workflows.
- Security and Data Privacy: Robust measures to protect sensitive data throughout the annotation process.
Keymakr: Leading the Charge in Image Annotation Solutions
Keymakr stands out as a premier provider in the realm of software development focused on image annotation for machine learning. Our platform combines cutting-edge AI assistance, user-friendly interface, and comprehensive quality control measures, empowering businesses to accelerate their AI initiatives with confidence.
With extensive experience in deploying large-scale annotation projects across diverse industries, Keymakr emphasizes adaptability, precision, and scalability. Our solutions integrate seamlessly with your AI development pipeline, reducing time-to-market and optimizing data quality for superior model performance.
Conclusion: Embracing the Future of AI with Superior Image Annotation
In the digital era where data-driven decision making dictates success, image annotation for machine learning emerges as the cornerstone of effective AI systems. From autonomous vehicles to healthcare diagnostics, the impact of precise and efficient data labeling is profound and far-reaching.
By investing in advanced annotation tools, partnering with experienced software developers like Keymakr, and implementing best practices, businesses can unlock the full potential of their AI initiatives. The future belongs to those who prioritize data quality, innovation, and scalability in their image annotation strategies.
Empower your AI projects today with superior image annotation solutions and stay ahead in the competitive landscape of technological innovation.